Strengthening the Toolkit for Tax Compliance Management: Machine learning 1
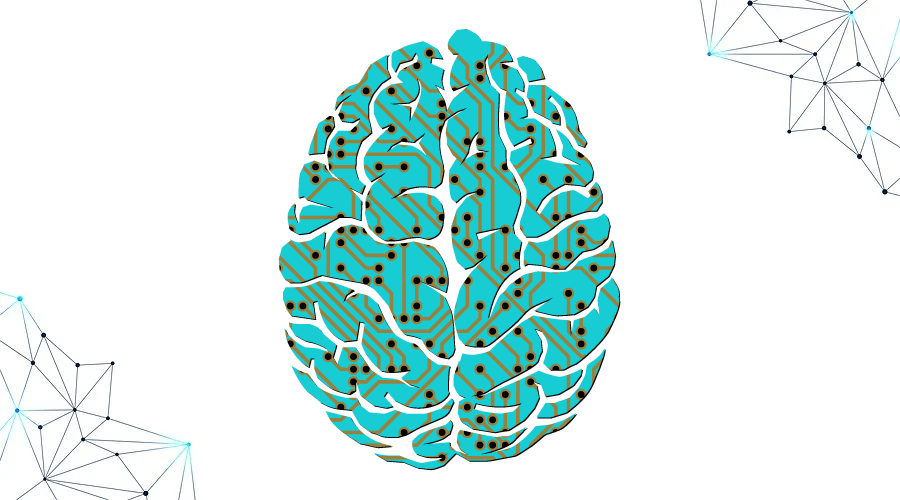
Intuitively
Even if we have been trained and specialized in tax administration matters, tax risk analysis, or matters of international taxation or special evasion practices in specific economic sectors such as tourism or mining, or in the management of processes for tax administration, including very rigorous field audits, perhaps the first thing that comes to mind when we hear concepts such as Artificial Intelligence or Machine Learning are notions of science fiction or futuristic movies. some of them quite obscure, in which humans are dominated or annihilated by robots, and others even funny in which we are usually ingratiated and usually outdone in tasks and activities of different intellectual or manual magnitude.
In a more specific area, you will have heard and read in various sources of information about different services and utilities based on the exploitation of technological devices designed and built with the help of ‘Machine learning’ techniques, including routines that classify your emails as ‘spam’ or ‘non-spam’, or that analyze images to suggest that you have a high – hopefully low – probability of some health anomaly or technological utilities that simply suggest words and expressions in advance as you write a document. No doubt there is a long list of examples today. Thus, it is possible that in the sphere of the tax administration you have heard ideas such as ‘the personal risk classification estimates the willingness to comply with tax obligations on the part of the 5 million taxpayers in the tax system’, or ‘the technological solution that interacts with taxpayers based on learning algorithms is equivalent to the work of 350 people’, or ‘the risk analysis model predicts potential cases of tax fraud allowing to treat the VAT chain before the occurrence of those frauds’ o ‘the imaging system recognizes high value goods within a property allowing a valuation of the property and enabling more accurate tax calculations’. These examples are striking, interesting, suggesting a new or imperative performance in the tax field, which may even excite you – or force you – to start a project in the short term that uses Machine learning techniques and related components such as digitalization, automation, blockchain and clouds, among others.
I do not intend to give a definition of the concept and even less about its possible scope – there are good specialists for this – although it can be observed that machine learning consists of:
Processing a lot of data from past experiences originating from a relevant activity or practice (a tax process) to feed and train statistical mathematical algorithm or learning models that make it possible to predict a future result or performance (of that tax process) obtaining improvements in that activity or practice (and new data which in turn are reused to re-feed-train such models, generating new information gains) in a permanent iterative process.
Based on this observation, a second intuition is that Machine learning can be seen as a technical concept, as technical as the concept of ‘Permanent Establishment’ in the agreements to avoid international double taxation, or ‘Correlative Adjustment’ in matters of transfer pricing, or as the accepted methods of valuation of assets and companies, such as ‘Multiple Companies’ or ‘Options’, or the ‘Tax Process Matrices’. So Machine learning involves techniques as much or more rigorous than those disciplines precisely because of its mathematical statistical heart. Likewise, Machine learning should not be equated to concepts such as digitalization or automation, although it is possible to use those components (and vice versa) and other related ones to be able to exploit those predictions in the different stages of the tax process.
An additional intuition is that this difference in technical disciplines does not prevent the tax administrator from taking a look at the uses that can be made of the different technical possibilities that would be feasible when counting on the use of Machine learning, thus increasing the capacities contained in his Toolkit for tax compliance management.
In the past, this Toolkit for tax compliance management has been nourished by the work of collaborators and human capital with risk models, digitized and automated electronic invoicing devices, electronic VAT withholding devices, or with mechanisms for traceability of special consumption taxes based on marks or stamps and even with technological devices that allow offering taxpayers a pre-filled VAT or Income proposal, all of which constitutes a wide range of possibilities and capacities to structurally influence the country’s tax compliance levels.
Another intuition relates to whether these Machine learning-based artifacts that would allow for predicting future results and improving performance on that basis are working globally. Undoubtedly, the top management of the Tax Administration needs support to embark on such a technical endeavor. Although there are numerous OECD and CIAT reports that are qualitatively blowing with the wind, it seems appropriate to comment on some real cases of use based on Machine learning that may guide the different exploitation possibilities that the tax administrations may undertake.
Some examples of use
– Advanced deep analysis model with communication system with taxpayers
The Australian Tax Administration (ATO) has managed to implement a group of tools aligned with Machine Learning techniques to analyze the tax system in real time, detect anomalies and treat them with automated tools that interact with taxpayers. This then implies that the techniques and possibilities include the stages of risk analysis, treatment selection and communication and mitigation of risks or breaches at a distance with the taxpayers.
In fact, as far as risk analysis is concerned, the ATO’s technology kit uses supervised analysis models with ‘k-type nearest neighbor’ and ‘neural network’ algorithms that detect anomalies, which characterize the behavior of the taxpayers, predict risk cases and propose them for treatment. To perform the remote or virtual treatment, the kit pushes taxpayers to make choices, for example, to claim that their costs or credits are correct or within an acceptable range.
One specific application of the above has been reported by the ATO on expenses declared for income tax purposes. For the 2017 tax year alone, based on interactive business and support robots, the “close neighbors” of 3.3 million taxpayers were analyzed, and of these, 230,000 taxpayers were asked to review the reasonableness of the expenses deducted in the income tax return.
– Model for predicting income and income tax deductions
In 2017 the Norwegian Tax Administration’s SKATT developed a ‘Proof of Concept’ to automatically generate virtual proposals for tax deduction using Machine Learning.
The model considered a traditional dataset of the tax administration and the development of a Machine learning routine that allows to predict (or estimate) the level of income, its composition, the level of debts and the family situation of the individual taxpayer in order to establish the origin of the legal deductions in the annual income tax return.
This artifact of Machine Learning also considered the use of classification models based on ‘sentimen analysis algorithms’, or ‘feeling analysis algorithms’ that perform text analysis and natural language processing available in social networks to classify words or phrases as positive, negative or neutral.
During the development of the proof of concept, different algorithms typical of Machine learning were used:
A reported lesson was that the separate use of these models was discarded early because the individual results would not have been satisfactory due to the high level of accuracy required when dealing with specific tax liabilities.
Therefore, the Norwegian SKATT set out to create an ‘assembled model’ in house with two specific focuses: one aimed at predicting who is entitled to deductions and the other to establish the amount of the deductions. With this assembled model, it would have been possible to reduce the impact of variables with low presence in the datasets, particularly those related to deductions. The assembled model considered utilities from “neural network”, “random forest”, “gaussian processes” and “two stage random forest” algorithms.
One of the warnings left by this proof of concept was that there was no way to establish what was going on inside the assembled model, i.e., a staff member alone was not able to monitor and explain the results of the model. The proof of concept established that the model correctly predicted the income level in 86% of the cases and in the case of deductions in 76% of the cases.
– Model for detecting changes in residence and jurisdiction
Also in 2017, SKATT Norway formulated a Machine Learning model so that it will automatically detect Norwegian residents who have emigrated from the country without notifying the tax administration and the central government.
Usually, migration controls are manual and it is almost impossible to control the tax situation of migrants, which is relevant for the application of the principle of global income and conventions to avoid double taxation, including the mitigation of potential abuse schemes, all in a context of European Community law and its four freedoms.
For the development of the test, SKATT databases, the National Register of Persons databases, debt databases and a model of home-made machine learning with the help of an external company were considered. The process of the model yields a list of people who should be further investigated through different communication channels.
In total, about 200 anonymized variables were established for pre-processing, of which these four had the highest weight according to the decision tree algorithm:
The test model achieves 68% confidence in identifying those who did leave Norway (true positives) and 99.5% confidence in those who have not left (true negatives). This proof of concept ended with a list of 23,000 people whom the model estimates would have left Norway without paying their annual taxes.
– Model for estimating the value of real estate
This is a model of valuation of the market value of real estate for a county or department that has about 400 thousand properties with high rates of urbanization of new housing, educational and industrial projects, where the human capacity to update the value of real estate based on traditional models is exceeded by the explosive growth of that territory.
The process considers delivering, on a daily basis, datasets from the county to the external company so that the Machine learning model indicates the estimated value of the properties. This means that the different municipal, real estate, notary, commercial and banking processes are providing information which is cleaned, catalogued and pre-processed on a daily basis based on automated routines.
One of the views generated by the model consists of a georeferenced map that allows visually determining which properties show a value that would be out of range considering the daily data inputs received. This then allows for further analysis or preventive treatment action on these properties.
Although this example is not directly related to the performance of tax administrations, it serves to highlight the possible uses in tax management, for example, in the calculation of excise taxes on large fortunes or on high-value real estate, which is interesting considering the usual pressures on these segments by social groups of citizens.
To be continued…
I consider it highly advisable that Machine learning-based artifacts begin to be seen in the region’s tax administrations as one more type of analysis, treatment and communication actions with taxpayers in an analogous manner to the examples summarized in the previous use cases.
In fact, in these times of the fourth revolution, it is a personal duty of the directors of the Tax Administration to know and specify how to strengthen the Toolkit for the management of tax compliance using different resources such as Machine learning and related technological components, matters that we will keep commenting in additional blogs.
4,829 total views, 3 views today
1 comment
Thanks for providing information about taxes in this blog and more about other information also we also do the same work of tax consultants